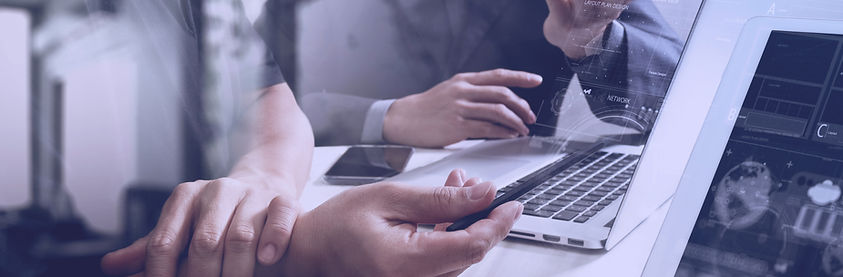

Agent-Based Modelling Technology
What is Agent-Based Modelling?
An agent-based model (ABM) is a computational model for simulating the actions and interactions of autonomous agents (both individual or collective entities such as organizations or groups) in order to understand the behavior of a system and what governs its outcomes. ABM is an alternative to equation-based models (regressions, SEM etc.). ABM allows, for example, making reasonably realistic digital copies of every citizen, organization and building in a municipality, and then run a simulation based on the intuitively understandable interaction rules.
Equation-based (socioeconomic) models, such as regressions build by national statistic offices, are well-established and widely accepted. They deliver robust predictions based on large samples. ABM is broadly used in natural science, but it gradually finds it’s way to the socioeconomic field. This development happens mainly due to better access to open data and growing computational power available to practitioners.
ABM's main advantages compared to equation-based methods are:
-
Ability to predict tipping points and emergence (new effects that are not reducible to sum of the parts of the original data),
-
Relatively easy integration of network effects, learning and adaption of individuals,
-
Relatively easy to account for individual decision making and bounded rationality,
-
Relatively easy to handle heterogenic agents,
-
ABM works at individual and local level and it is less dependent om large samples,
-
ABM represents people as individuals rather than aggregated populations or functions. It is important for empowerment and communication of results to end-users.
Areas of practical application
Agent-based models are used by the R&D departments in the industrial sector - ranging from optimization of chemical reactions to development of automated driving systems.
There are also examples of consulting firms applying ABM to social and economic tasks:
-
KPMG has simulated Melbourne population and travel behavior: https://www.youtube.com/watch?v=j6aPe0sYvTY
-
PwC has developed a Covid-19 Simulator: https://www.pwc.at/en/covid19-information-and-preparation/covid-19-simulator.html
-
Deloitte has published a report titled “Agent-based modelling for central counterparty clearing risk”: https://www2.deloitte.com/content/dam/Deloitte/uk/Documents/audit/deloitte-uk-modelling-ccp-resilience.pdf
-
The patterns of the users (tourists, citizens, workers) affecting the energy consumption in Andorra (with General Electric): https://www.media.mit.edu/projects/andorra-energy-environment/overview/
-
Modelling UK’s heat market report: https://nic.org.uk/app/uploads/frontier-nic-agent-based-heat-model-stc-100521-v10.pdf
​
Research
Embodying ABM into any socioeconomic research is not (yet) a mainstream, rising the interest from the journal editorial teams that is increasing the chances for a publication in prestigious journals. Our team can refer to the publication in, for example, top tourism research journal (Vinogradov, E., Leick, B. & Kivedal, B.K. (2020). An agent-based modelling approach to housing market regulations and Airbnb-induced tourism. Tourism Management, 77).
ABM is “a form of computational modelling whereby a phenomenon is modelled in terms of agents and their interactions”, where an agent is defined as “an autonomous computational individual or object with particular properties and actions” (Wilensky & Rand, 2015, p. 1).
In the last 20 years, the convergence of different factors - the rise of the complexity of science, the “data deluge” and the advances in information technologies - triggered a paradigm shift in the way we understand complex social systems and their evolution. Beyond shedding new light onto social dynamics, the emerging research area of Computational Social Science (CSS) is providing a new rationale for a more scientifically-grounded and effective policy design (Dabbaghian & Mago, 2014; Jackson, 2014; Lettieri, 2016; Levitt, 2012). From a theoretical and epistemological point of view (Benthall, 2016; Goebel, Siekmann, Wahlster, & Squazzoni, 2009) CSS, the “integrated, interdisciplinary pursuit of social inquiry with emphasis on information processing and through the medium of advanced computation” (Cioffi-Revilla, 2010), is grounded on a scientific perspective in which different research traditions flow into one.
The two main simulation techniques used today in social and organizational research are Systems Dynamics (Forrester, 1961) and Agent-Based Models (ABM) (Hamill & Gilbert, 2015). System Dynamics (SD) models are based on the idea that the evolution of a social system can be represented as the result of complex cycles of action and feedback which can be described in mathematical terms. On this assumption are grounded simulations in which the phenomenon under investigation is represented as a set consisting of variables (stocks) and rates of change (flows) associated with them. Today, these models are the basis for many applications in the industrial sector and social sciences. Agent-Based simulation Models are led by the theoretical assumption that the macro-level social phenomena are the result deriving from the interactions occurring, at the micro- and meso-level, between individuals (persons, organizations, institutions), and between individuals and the environment. ABMs typically include a set of heterogeneous artificial agents simulating real-world actors and their behaviors, a set of rules of interaction and an environment in which dynamic, organizational and spatial characteristics are defined. Social and organizational simulation provides policy-makers with the ability to run a what-if analysis allowing to observe the effects potentially deriving from different choices by means of well-developed models of a given “target system” (Cioffi-Revilla & Rouleau, 2010).